What is the p-value?
The p-value is a statistical measure of significance. Here are some links to explanations of p-values:
- The Rational Wiki page on statistical significance, which is not too bad. Pictures are handy.
- The Wikipedia page on p-value, which frankly is so confusing it may as well be discussing a prenuptial value.
- What a p-value tells you about statistical data, from the Dummies franchise.
They did not help me all that much besides providing a broad background on the topic, so I will try to clarify things further.
What is calculated when one calculates a p-value?
To understand p-values, it is important to have a concept of what one does when you calculate a p-value. You are testing a hypothesis or a conjecture - a theory in layman's terms. A theory has far more evidence than a hypothesis in a scientific context, so I will not be employing that terminology here.
You are comparing two hypotheses. You are comparing your alternative hypothesis to the null hypothesis. The hypothesis that you are testing when you calculate the p-value is the null hypothesis, and not the alternative hypothesis. This is where the confusion sets in.
How can this be simplified further?
Let's forget about complex models with many variables and just focus on one linear regression: Salary and work experience. We may formulate a research question as follows: Is there a relationship between work experience and salary?
- The null hypothesis is the claim that our hypothesis is false. In his case, the null hypothesis is the assumption that there is no relationship (there is no effect, or there is a null effect) between work experience and salary.
- The alternative hypothesis is the claim that the null hypothesis is false. In this case, it is the assumption that work experience influences salary, or that there is an effect. If there is not a null effect, there is an effect.
Why are we testing the null hypothesis?
Because we are using a logical technique called reductio ad absurdum. This means that we are trying to prove that the null hypothesis is false by showing it would be completely absurd if it were true, because the correlation between work experience and salary is so strong. This technique is an attempt to falsify the null hypothesis. In other words, we are trying to reject the null hypothesis.
If we can't prove the null hypothesis false, we assume that it is true. And what is the null hypothesis? 'There is no relationship between salary and work experience', in this case. So we are trying to prove that it is false that there is no relationship between salary and work experience.
What do p-values tell us?
The higher the p-value, the more likely that our null hypothesis is true, and that our alternative hypothesis is false. The lower the p-value, the more likely that our null hypothesis is false, and that the alternative hypothesis is true. But again, we are testing the null hypothesis, not the alternative hypothesis.
If we have a low p-value, that means 'how likely do I think that the null hypothesis is false?', and this is where we are committing terrible sins because it's not a probability measure for how false or true a given hypothesis is.
Rational Wiki has a good discussion on how p-values relate to probability. It is in the section alpha value versus p-value on the statistical significance page.
What about more complex models with more than two variables?
For a more complex model, our null hypothesis could be for example: 'spending on r&d, spending on marketing and ...(however many possible effects we may have), have no effect on company profit'.
The alternative hypothesis for this more complex model would be: 'spending on all these things does correlate with company profit'.
Different combinations of variables may be chosen to construct the null hypothesis, which naturally would affect the opposing alternative hypothesis. This is the idea behind backward elimination of models with p-values. Our p-value tells us which null hypothesis is the most unlikely, so it would have the highest p-value. This means that we assume the alternative hypothesis for which the null hypothesis has the lowest p-value.
In Summary
A p-value is a measure of statistical significance. It can be thought of as how likely your null hypothesis true. A low p-value means your null hypothesis is unlikely, and null hypothesis means 'there is no effect' or 'there is null effect'. If you reject that, it means you assume the alternative hypothesis, namely that there is an effect. A lower p-value means more faith in the alternative hypothesis. A higher p-value means more faith in the null hypothesis.
@mathowl I want to catch them all.
Content type: Eductional, long
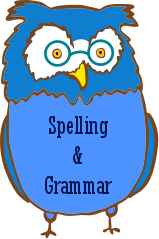
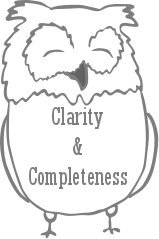
Awarded 4 out of 6 owls:
Details: The education sensation owl was not awarded since the example could be more entertaining. The originality owl was not awarded since it requires the math to be explained in a novel/creative way.
Note: the post was not upvoted since it is more than 7 days old.